‘It’s going to set the bar pretty high’: A path forward for trusted AI in breast cancer risk prediction
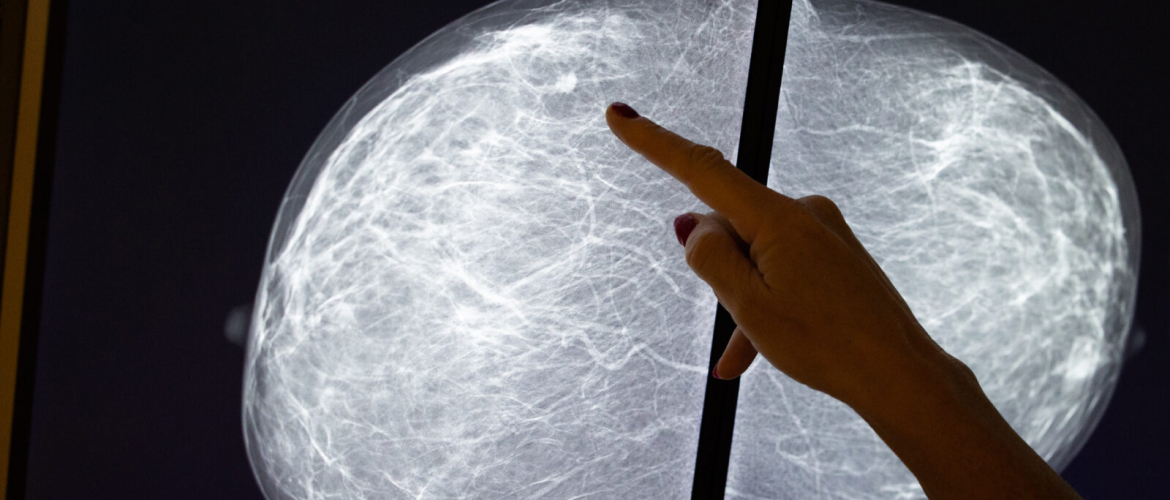
The rise of AI in healthcare presents challenges around equitable care as new tools are trained on existing health records, which could lead to perpetuating biases in current standards of care. Researchers at MIT Jameel Clinic are sensitive to this challenge. As the team develops its AI early-detection breast cancer model, Mirai, the technology's algorithm is being trained and tested across a diverse set of patient data to ensure its effectiveness on a wide range of patients. According to a paper published in the Journal of Clinical Oncology, the model is being tested at seven hospitals around the world, including Brazil, Sweden, Taiwan, Israel, and the US, where the model first introduced at Massachusetts General Hospital.
Testing across a wide set of geographies is important, but painstaking and rare. Adam Yala, a PhD student at MIT working with Regina Barzilay, MIT Jameel Clinic's faculty lead for artificial intelligence, described some of the challenges associated with securing collaborators and accessing and analysing patient data from the various facilities. Due to regulations in Taiwan's Chang Gung Memorial Hospital whereby patient data is required to remain at the hospital's physical location, Yala traveled to Taipei. 'The only way to validate Mirai on their data was to physically show up, directly login into a machine and do the work on premise,' he noted.
Collecting and analysing these diverse data sets is a worthwhile process that has shown encouraging results in the Mirai's development. Results from Emory University showed Mirai performed similarly across racially distinct patients, which is a crucial indicator in a country where Black women are significantly more at risk of death from breast cancer.
As the team moves to testing Mirai in prospective clinical trials, it plans to make Mirai's code freely accessible to identified hospital systems to enable hospitals to conduct their own studies for local validation ahead of deployment. The team will continue to tackle questions around translating algorithmic risk calculation into effective clinical practice.
'All of our health care systems already capture the biases in some way or another, so how we effectively build systems off generally biased data is a really open challenge,' explained Yala. 'How do you build technologies while making it so that what it is predicting is not regurgitating the past? Finding the thing that's actually going to drive an equitable improvement is an open technical problem. But it's one we can study and we can solve.'