How an archeological approach can help leverage biased data in AI to improve medicine
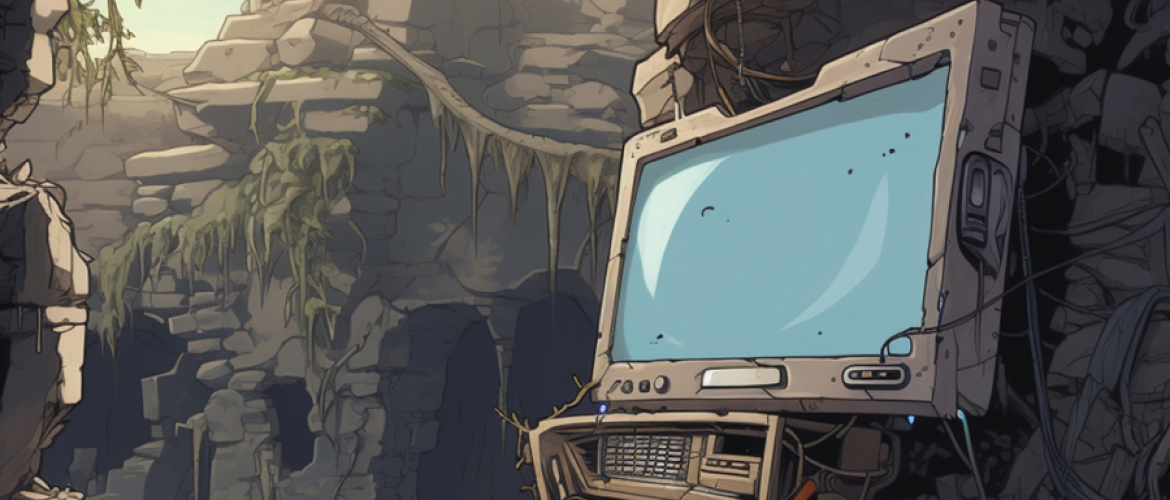
Marzyeh Ghassemi, 2022-2023, has co-authored an opinion piece with Kadija Ferryman and Maxine Mackintosh on AI data bias. 'Considering biased data as informative artifacts in AI-assisted health care' was published in the New England Journal of Medicine. The paper's authors suggest that rather than viewing biased clinical data in AI health care models as a hinderance, an archeological approach to the data may reveal valuable information about the belief systems, cultural values and practices that have led to inequities in health care.
Marzyeh speaks to the inspiration for the paper. "We had used analogies of data as an artefact that gives a partial view of past practices, or a cracked mirror holding up a reflection. In both cases the information is perhaps not entirely accurate or favourable: Maybe we think that we behave in certain ways as a society — but when you actually look at the data, it tells a different story. We might not like what that story is, but once you unearth an understanding of the past you can move forward and take steps to address poor practices," she says.
While the paper's authors propose that there is much to be learned from biased data sets, they note that fixing biased algorithms and generating better and more robust data remains essential to the progress of AI-assisted healthcare.
Marzyeh speaks about the National Institutes of Health (NIH)'s approach, saying the agency has “prioritised data collection in ethical ways that cover information we have not previously emphasised the value of in human health — such as environmental factors and social determinants. I’m very excited about their prioritisation of, and strong investments towards, achieving meaningful health outcomes.”
While she understand that the public remains concerned about the ethical implications of AI-assisted health care, Marzyeh tells individuals, "you shouldn't be scared of some hypothetical AI in health tomorrow, you should be scared of what health is right now. If we take a narrow technical view of the data we extract from systems, we could naively replicate poor practices. That’s not the only option — realising there is a problem is our first step towards a larger opportunity."